More than ever, today’s youth are turning to video games as a primary entertainment outlet (let’s be real… some adults, too). According to the Entertainment Software Association (ESA), nearly 2/3 of all U.S. households have at least one “frequent gamer.” And, why not accompany the mayhem created during three hours of mazing around in a paradoxical Skyrim world with a little Steve Vai thrashing his guitar in the background. It could be assumed that the more a smart, youthful individual tends to incorporate WoW or Halo into his or her lifestyle habits, the more likely he or she is to educate the neighbors by blaring some John Petrucci through an open window, turned all the way up to 11. A clear correlation.
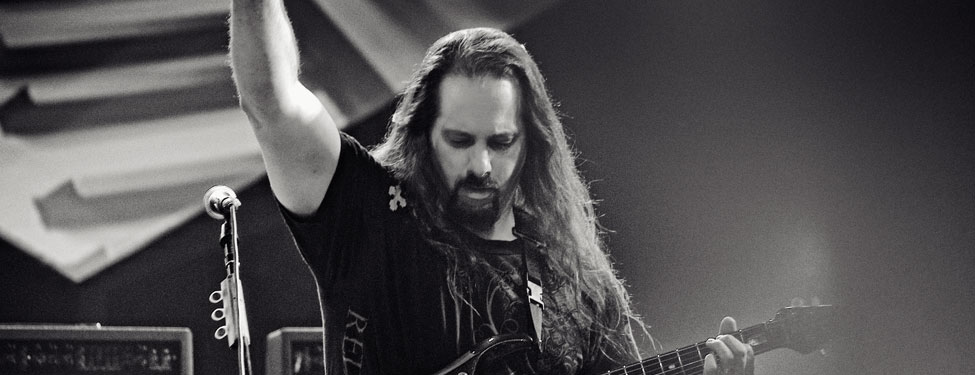
John Petrucci, © 2016 Dunlop Manufacturing, Inc
Or not? Do we actually know if musical preference and hours spent gaming are associated? And further, does one cause the other… do entertainment habits affect musical taste, or vice versa? Can we even put a direction of influence on it? It is a common mistake to confuse correlation with causation. Ice cream sales increase in the summer, and so do the number of heat-related deaths, but that does not mean if you eat enough hand-churned raspberry chip your risk of fatality by fever increases. They are not associated, let alone causative. So, how do you distinguish the two, and how can you tell when correlation does prove causation?
Correlation: A representation of the mutual relationship that exists between two variables; as one changes, the other is affected. Examples:
- A relationship exists between sedentary time and all cause mortality. Cigarette smoking is correlated with the development of emphysema.
A relationship can be positive (also called direct, where both variables increase or decrease in the same direction), or it can be negative (also called indirect, where the variables have opposite effects on each other). However, correlation does not imply causation.
Causation: One variable influences a change in a second, associated variable. They are correlated – a basic requirement. The measurement of an independent variable “A” may lead us to a predictable verdict with an associated dependent variable “B.” A few examples: Increases in sedentary time may cause an increase in blood pressure. A decrease in cigarette smoking also decreases the risk of developing atherosclerosis.
More often than not, causation may be interpreted, yet it must be noted that several variables may be responsible for promoting an expected outcome. Research has shown that increases in both smoking and poor dietary habits also lead to increases in blood pressure – sedentary time is not the sole influence. How can we clarify relationships between variables and establish a justification for causality?
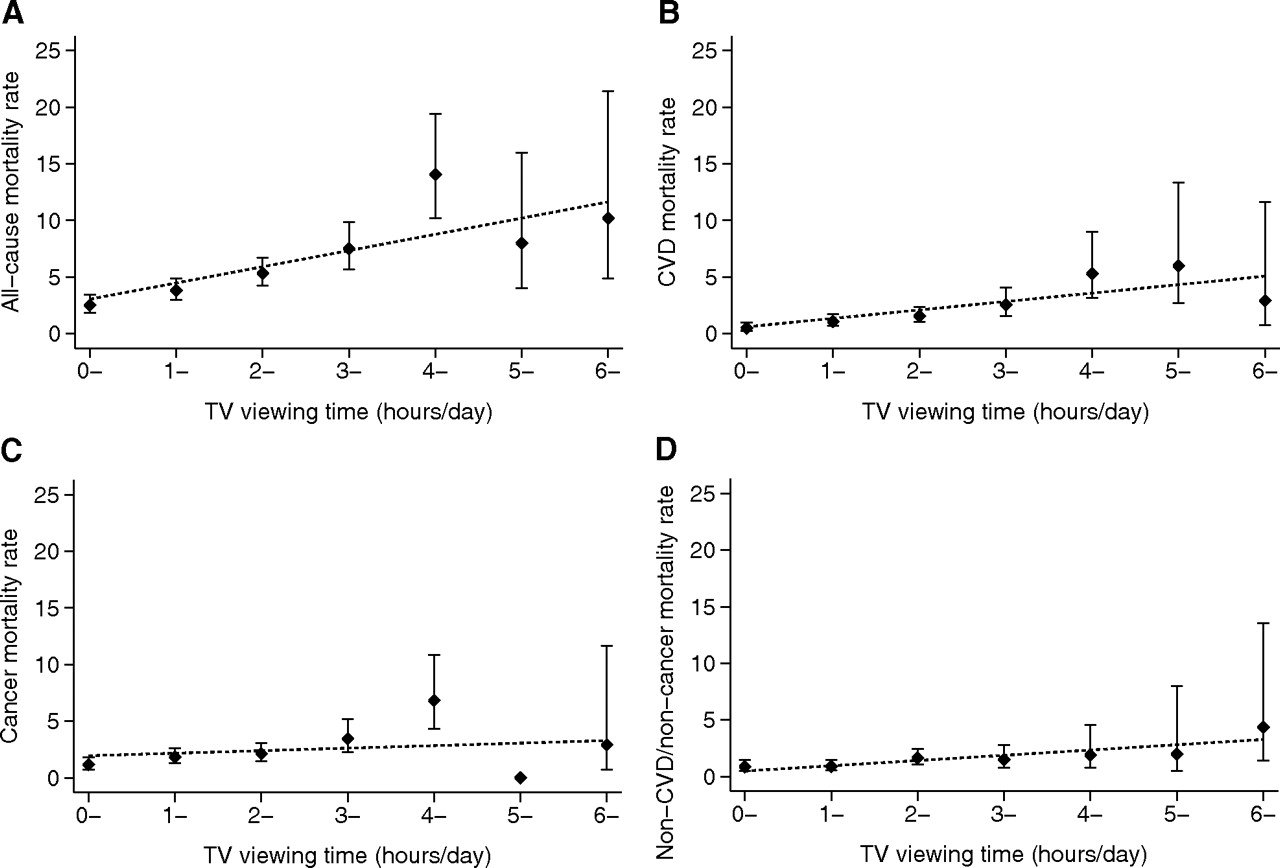
Dunstan, et al., 2010. Each 1-hour increment in television viewing time was found to be associated with an 11% and an 18% increased risk of all-cause and CVD mortality, respectively. The figure here: unadjusted all-cause (A), CVD (B), cancer (C), and non-CVD/noncancer (D) mortality rates per 1000 person-years according to television (TV) viewing time (h/d). Dashed line presents the linear relationship between increments of television viewing time and each respective mortality rate.
Sir Austin Bradford Hill
In 1965, Sir Austin Bradford Hill of London, England, published a foundational document outlining nine essential criteria used to establish causation between associated variables. A British epidemiologist and notable professor of medical statistics, Hill became well-known in the medical field for determining the causative relationship between smoking and lung cancer rates. His approach to research has been institutionalized, becoming a must-know for all scientists involved in study design and analysis.
Hill’s Criteria for causation – analyze the following:
- Strength of the association. How much existing research supports the claim – is there strong evidence? Does cigarette smoking increase the risk of lung cancer? Yes. Heaps of research support this (LINK to meta analysis). Does the elimination of gluten really prevent digestive upset, fatigue and depression? For the overwhelming majority, NO. Read our article. Stop believing all the dietary rubbish.
- Consistency. Are research outcomes repeatable? If consistency exists between the majority of study results, the higher the likelihood a causative relationship exists.
- Specificity. Is an associative cause able to be pin-pointed? Does a specific population, environment or other variable particularly increase the rate or prevalence of the outcome? Couple specificity with strength – the more magnanimous the strength of a specific influence – a causative relationship may be assumed with little hesitation.
- Temporality. Is there a clear direction – a cause and an effect? Does A cause B and not the other way around? The relationship should be unidirectional.
- Biological gradient. Is there a dose-response relationship? Do the independent variable “A” and dependent variable “B” have a linear relationship? Higher doses of oxycodone increase rate of drug dependency. Increases in sedentary time also increase risk for developing cardiovascular disease.
- Plausibility. Basically, does the relationship make sense – could it be possible? This criterion relies on what we know today. Remember, science always changes.
- Coherence. Does the relationship fit within what is stated in the current scientific literature?
- Experiment. A quality experiment may provide strong evidence for causation. The randomized controlled trial (RCT) is considered a gold standard.
- Analogy. Similar associations exist elsewhere. This criterion is considered least likely to help when determining causative relationships.
So, just because you are a beautiful, career-oriented woman with a history of terribly failed relationships, does not mean you will inevitably be donned Queen Cat Lady… whew!
Fundamentally, what we determine today may be displaced by new discoveries in the future. This is the wondrous personality of science. Even when causative associations are deduced and proclaimed, uprooted and re-proclaimed, habits are difficult to manipulate. It could be safely stated that the human psyche does not like change. Diets, political preferences and other valued self-identifiers are synonymous to religion: deep-seeded and somewhat emotional attributes to societally-connected individualism. Nonetheless, exercise still reduces risk of stroke, and regular exposure to ultraviolet radiation escalates the risk of developing melanoma. Keep your mind malleable. Being smart is not only understanding the difference between correlation and causation, but acting on best judgment.
References:
Dunstan, D. W., Barr, E. L. M., Healy, G. N., Salmon, J., Shaw, J. E., Balkau, B., … & Owen, N. (2010). Television viewing time and mortality the australian diabetes, obesity and lifestyle study (AusDiab). Circulation, 121(3), 384-391.
Field, A. (2009). Discovering statistics using SPSS. Sage publications.
Hill, A. B. (1965). The environment and disease: association or causation?. Proceedings of the Royal society of Medicine, 58(5), 295.
Salkind, N. J. (2016). Statistics for People Who (Think They) Hate Statistics: Using Microsoft Excel 2016. Sage Publications.